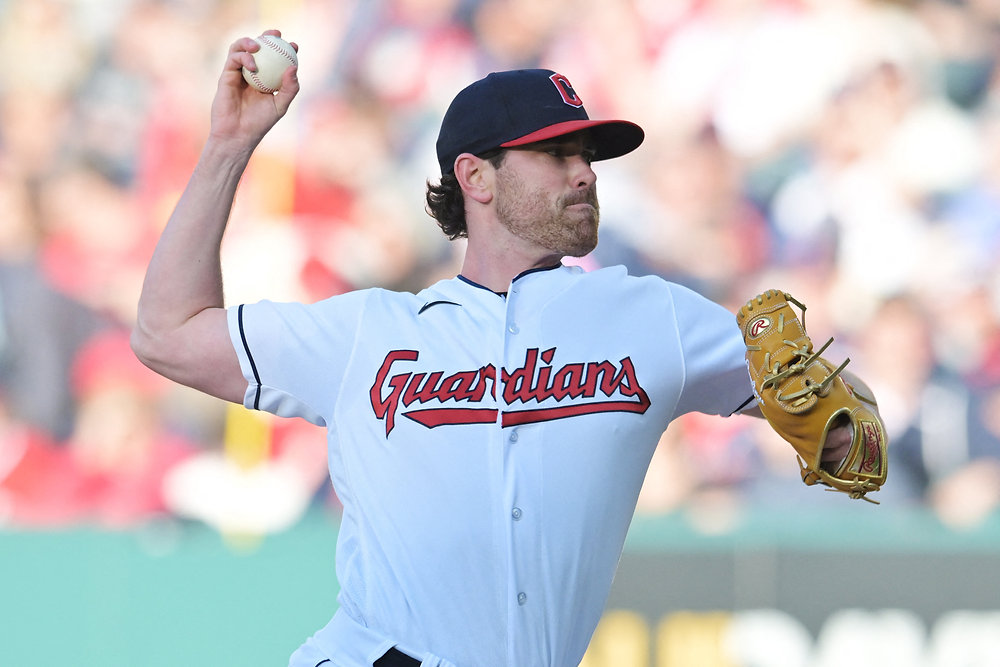
Shane Bieber pitching for the Cleveland Guardians, CCed by Liscense 2.0
Pitch tunneling is one of the many hot terms thrown around the baseball space. Analysts, players and coaches, commentators, and fans alike all seem to have different opinions about its effectiveness as well as its legitimacy as a whole, but what is pitch tunneling anyway? Although sometimes made to seem like a dark art or an advanced science concept, pitch tunneling at its core isn’t all that complex, and is simply another way for pitcher’s to deceive hitters. In this article, I’ll be examining whether or not pitch tunneling actually works, and if so, how much.
Pitch tunneling is the concept of matching pitched balls’ flight paths closely enough to make them appear to be the same pitch long enough to fool the batter before they move in different directions. This may be confusing to visualize on your own, so here’s a video of Shane Bieber performing some tunneling, courtesy of Pitching Ninja. There are several factors that go into tunneling 2 pitches together; the most important being release point, pitch trajectory, and plate location or where the pitch ends up. As tunneling relies on 2 pitches traveling the same path and then breaking in different directions, it would be impossible to tunnel 2 pitches released from 2 different arm slots. However, even if 2 pitches are released from the exact same point, it does no good if one is a spiked curveball and the other is a fastball that sails over the catcher’s head, so the 2 pitches must also travel relatively similar paths for as long as possible to deceive the hitter. Furthermore, if the 2 pitches don’t end up doing anything differently movement wise, then that also defeats the purpose of the pitches being tunneled, as the ball ends up in the same place no matter which pitch is thrown.
This all seems pretty awesome at first glance. Throw 2 pitches down the same path and the hitter is completely fooled right? Well maybe if all pitchers were robots and were capable of doing that everytime then yes, pitch tunneling would be pretty foolproof. However even in the big leagues, no pitcher hits his spot anywhere near everytime, and even if they did, a lot of times hitters simply sit on certain pitches based on that pitcher’s tendencies and will take anything that isn’t what they’re looking for. In addition, 2 pitches could be perfectly tunneled but be balls, which again defeats the purpose of the practice. So does pitch tunneling actually work outside of theory? Let’s answer that question with a little bit of statistical analysis.
First, we need a metric to measure how well 2 given pitches are tunneled. As I said earlier, pitch tunneling mainly boils down to 3 factors: release point, flight path, and plate location. A great example of a basic metric combining these 3 factors is the Tunnel Score which is outlined in this great article. Tunnel Score takes into account the difference in release point between 2 pitches by calculating the euclidean distance between the 2 points of release. A higher distance will result in a lower score and vice versa. Tunnel Score then uses the ratio between the difference in location of the 2 pitches with movement and the difference in location of the 2 pitches without movement to see how similar they were early in their flight and how different they were at the end of their trajectories. This means that a lower difference location without movement and a higher difference with movement will result in a higher Tunnel Score and vice versa. With each factor accounted for, the whole formula for the Tunnel Score metric is: (actual distance tunnel distance) – release distance with actual distance being the difference in the pitch locations with movement and tunnel distance being the difference between the locations without movement.
There are 2 changes I have made from the original formula. The first being that I have chosen to measure actual distance and tunnel distance in inches instead of feet. This is because I believe that a smaller change in movement should be magnified in the tunnel score calculation, whereas release distance should still be measured in feet because a small change will have minimal effects on the tunneling effect of the pitch. The second change I am going to make is I am going to scale Tunnel Score to 100 to make it into Tunnel Score+, meaning that the average score will become 100, a score of 115 will be 15% above average, and a score of 85% will be 15% below average. This will not only make it easier to understand what is a good or bad score, but it will also make it easier to make further calculations later in the analysis.
Next, we need a metric to grade the quality of each pitch itself from a non-tunneling standpoint. For this we will simply use the run values of each pitch as provided by Baseball Savant. In doing this, we get a measure of how good each one of a pitcher’s pitches are by itself, not sequenced with another pitch. I am also going to scale the run values to 100 for each pitch type in order to put them on the same scale as Tunnel Score+.
To use these 2 metrics to grade the effectiveness of tunneling in adding value to the pitches that are sequenced together, I will first randomly select 100 pitchers with a minimum of 3 different pitch types thrown, and 200 pitches thrown this season. This will give me enough different combinations of pitch sequences as well as a group of pitchers selected without bias so that I will theoretically have some pitchers who tunnel very well, and some who don’t, as well as some who have great individual pitches and some who don’t. I will then calculate the Tunnel Score for each 2 pitch sequence they threw throughout the entire season, and get the run value of each pitch they threw this season. Then, to test the effects of tunneling on the value of the pitches, I will check the correlation between the sets of values, looking for a pattern indicating an increase in run value with an increase in Tunnel Score, or the other way around. Now it’s time to take a look at my results and see if tunneling had a positive effect on the value of the pitches.
My random selection of pitchers worked as intended, as I had some names like Jack Leiter and Kenta Maeda who have abysmal run values for their Fastballs, as well as some names like Zach Wheeler and Cade Smith, who had very high run values on theirs. The most common sequences of pitches were Fastball-Changeup, Sinker-Slider, Fastball-Curveball, and each of those pair’s reversed versions, with the highest Tunnel Scores coming from Sinker-Slider and Fastball-Curveball combinations. This makes sense, as each of those pitch type pairs (other than Fastball-Changeup) contain Fastballs and Breaking Balls which move in different directions entirely. The surprise, however, came in the final results of my analysis; the test of the correlation between the Tunnel Scores and Run Values. While I expected there to be a somewhat strong correlation between the 2, meaning that I expected successful tunneling to increase the values of the pitches tunneled, I found that there was actually almost no relationship at all between the 2 with the correlation between them being just 0.07. This means that no matter whether a sequence of pitches was tunneled very well or very poorly, the run values remained largely the same. So why is this?
There are a number of reasons why tunneling didn’t contribute much to changes in run value. For instance, it is very possible that the tunneling did work on some occasions but didn’t work on others, thus balancing out the relationship. As tunneling requires such fine command of both movement and release out of the hand, I find it hard to believe that even the best arms in MLB could do it consistently enough for it to make much of an impact. Another problem with tunneling is that it often results in multiple pitches being thrown in the same location repeatedly, which gives an advantage to the hitter, especially if they are able to figure out the sequence in which the pitcher is trying to tunnel his pitches. However, the biggest reason in my opinion why there wasn’t a strong correlation is that tunneling will ultimately never outweigh the quality of the pitch itself. Even if 2 pitches follow the same trajectory and then break away from each other, perfectly tunneled, if the pitches are slow, poorly located, or have poor individual movement profiles, then the values of the pitches will still be low.
In conclusion, I believe that while the concept of tunneling sounds great, it simply isn’t a feasible deception practice in terms of consistently being able to perform it at a high level and, even if perfected, will never be more important than the quality of individual pitches themselves. Obviously, more research will be done on tunneling by fans and professional organizations alike, but as of now there is no definite benefit in trying to perform it, and when it is done successfully, it is more likely a coincidental phenomenon than a repeatable pattern.